Unlocking Business Potential: The Role of Annotation Tools in Machine Learning
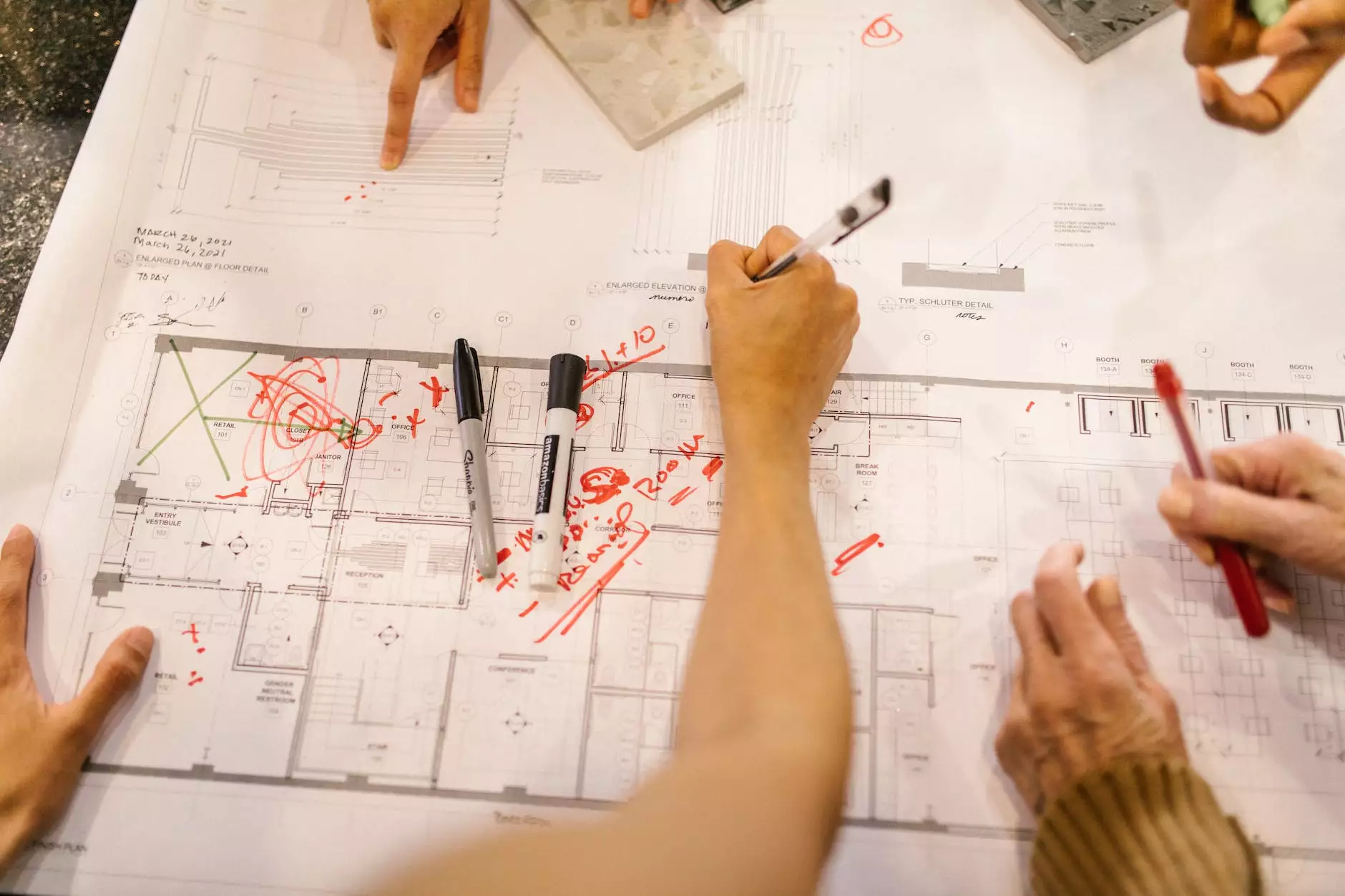
In today's digital landscape, the intersection of technology and business has never been more pronounced. Companies across various sectors are leveraging advanced technologies to streamline operations and enhance customer experiences. One such innovation is the use of annotation tools in machine learning, which plays a pivotal role in the rapid transformation within industries like Home Services, Keys & Locksmiths.
Understanding Annotation Tools and Their Importance
At its core, annotation refers to the process of labeling data, enriching it with information to make it useful for machine learning models. Annotation tools facilitate this process, allowing businesses to create high-quality datasets that drive accurate predictive models. In sectors that depend heavily on data, such as locksmithing—where data can inform everything from pricing models to customer service adjustments—annotation tools become invaluable.
What are Annotation Tools?
Annotation tools are software applications designed to assist users in labeling data effectively and efficiently. They can annotate various data types, including text, images, audio, and video. For businesses in the keys and locksmiths industry, this means they can leverage data to better understand customer behavior and refine their services.
Types of Annotation Tools
- Image Annotation Tools: Used for labeling images, facilitating tasks like object detection and image classification.
- Text Annotation Tools: Ideal for labeling textual data, important for sentiment analysis, chatbots, and more.
- Audio Annotation Tools: Useful for transcribing audio samples, essential for voice recognition applications.
- Video Annotation Tools: Crucial for tasks involving video recognition and analysis, such as security surveillance or customer behavior analysis.
Why Machine Learning is a Game Changer for Businesses
The machine learning revolution is reshaping how businesses operate. By harnessing vast amounts of data, companies can implement machine learning algorithms that learn and adapt over time, leading to improved decision-making processes. This section will explore key advantages of integrating machine learning into business operations.
Enhanced Decision-Making
With machine learning, businesses can make informed decisions backed by data-driven insights. For instance, a locksmith service can analyze past service calls and customer feedback to build a model that predicts peak times for work, helping them allocate resources more efficiently.
Automating Processes
Automation in business processes leads to increased efficiency and reduced operational costs. By utilizing machine learning, service providers in the home services industry can automate appointment scheduling, customer notifications, and even service follow-ups—all while improving the customer experience.
Personalizing Customer Experience
Machine learning enables businesses to personalize interactions with customers based on their preferences and behaviors. For example, a locksmith company can analyze customer data to send personalized promotions, enhancing customer retention and loyalty.
The Contribution of Annotation Tools to Machine Learning
The effectiveness of machine learning algorithms is heavily reliant on the quality of the data they are trained on. This is where annotation tools come in. They ensure that datasets are accurately labeled and comprehensive, paving the way for superior machine learning models. Below are some significant contributions of annotation tools.
Data Quality Enhancement
High-quality data is paramount for any machine learning initiative. Annotation tools allow businesses to consistently label data, minimizing the chances of errors that can compromise model performance.
Facilitating Diverse Applications
Organizations can use annotation tools to create datasets that cater to various applications within their operations, ranging from predictive maintenance in equipment for locksmiths to customer behavior analytics in marketing strategies.
Boosting Collaboration
Incorporating annotation tools fosters collaboration among teams working on machine learning projects. Multiple stakeholders—including data scientists, business analysts, and operation teams—can access and work on datasets, ensuring alignment and clarity in project objectives.
Implementing Annotation Tools in Business Operations
For businesses in the home services sector, particularly those specializing in keys and locksmithing, implementing these tools involves several critical steps:
Identifying the Right Tool
Choosing the appropriate annotation tool is essential. Factors such as ease of use, integration capabilities with existing systems, and support for various data types should be evaluated. Popular tools include Labelbox, SuperAnnotate, and Prodigy.
Training Staff
Investing time in training staff to effectively use annotation tools ensures that the data being labeled is of the highest quality. Training sessions can include how to identify what needs to be annotated and maintaining consistency in labeling.
Monitoring and Continuous Improvement
Once annotation tools are implemented, it is crucial to continuously monitor the quality of the labeled data and make improvements as needed. Regular assessments help identify any discrepancies and ensure the ongoing effectiveness of machine learning models.
Case Studies: Annotation Tools in Action
Examining real-world applications can provide valuable insights into how businesses can harness annotation tools effectively. Here are a couple of exemplary case studies:
Case Study 1: Keymakr's Transformation
Keymakr, a leading provider in the keys and locksmith services space, utilized annotation tools to enhance their customer service operations. By implementing a predictive model derived from customer data, they were able to forecast service demand more accurately, leading to optimized labor allocation and improved service times. Their customer satisfaction rates increased by over 30% within a year of implementing these solutions.
Case Study 2: Home Services Efficiency
A company specializing in Home Services, such as plumbing and electrical work, adopted annotation tools to streamline its process. By annotating historical service calls, they identified patterns in customer behavior, allowing them to adjust their marketing strategies for better targeting. This resulted in a 40% increase in lead conversion rates and a significant reduction in customer acquisition costs.
The Future of Annotation Tools and Machine Learning in Business
The future of business operations lies in intelligently harnessing data. As machine learning continues to evolve, the importance of annotation tools will only grow. Businesses that adapt and integrate these innovations will gain a competitive edge. The trend towards more automated, efficient operations is set to reshape industries.
Key Trends to Watch
- Increased Automation: Machine learning combined with annotation tools will lead to more automated decision-making processes in businesses.
- Enhanced Collaboration: Future annotation tools will likely include even more collaboration features, allowing teams to work together seamlessly.
- Integration with AI: Annotation tools may become more intelligent, utilizing AI to suggest labels based on previous annotations.
- Focus on Data Privacy: As data use grows, so will the emphasis on data privacy, leading to the emergence of compliance-ready annotation tools.
Conclusion
In summary, the integration of annotation tools in machine learning represents an exciting frontier for businesses, especially in the Home Services, Keys & Locksmiths sectors. By improving data quality, enhancing automation, and fostering collaboration, these tools not only optimize current operations but also unlock new opportunities for innovation and growth. Businesses that embrace these changes will be well-positioned for success in the rapidly evolving marketplace.
annotation tool machine learning