The Critical Role of Data Labelling Tools in AI Development
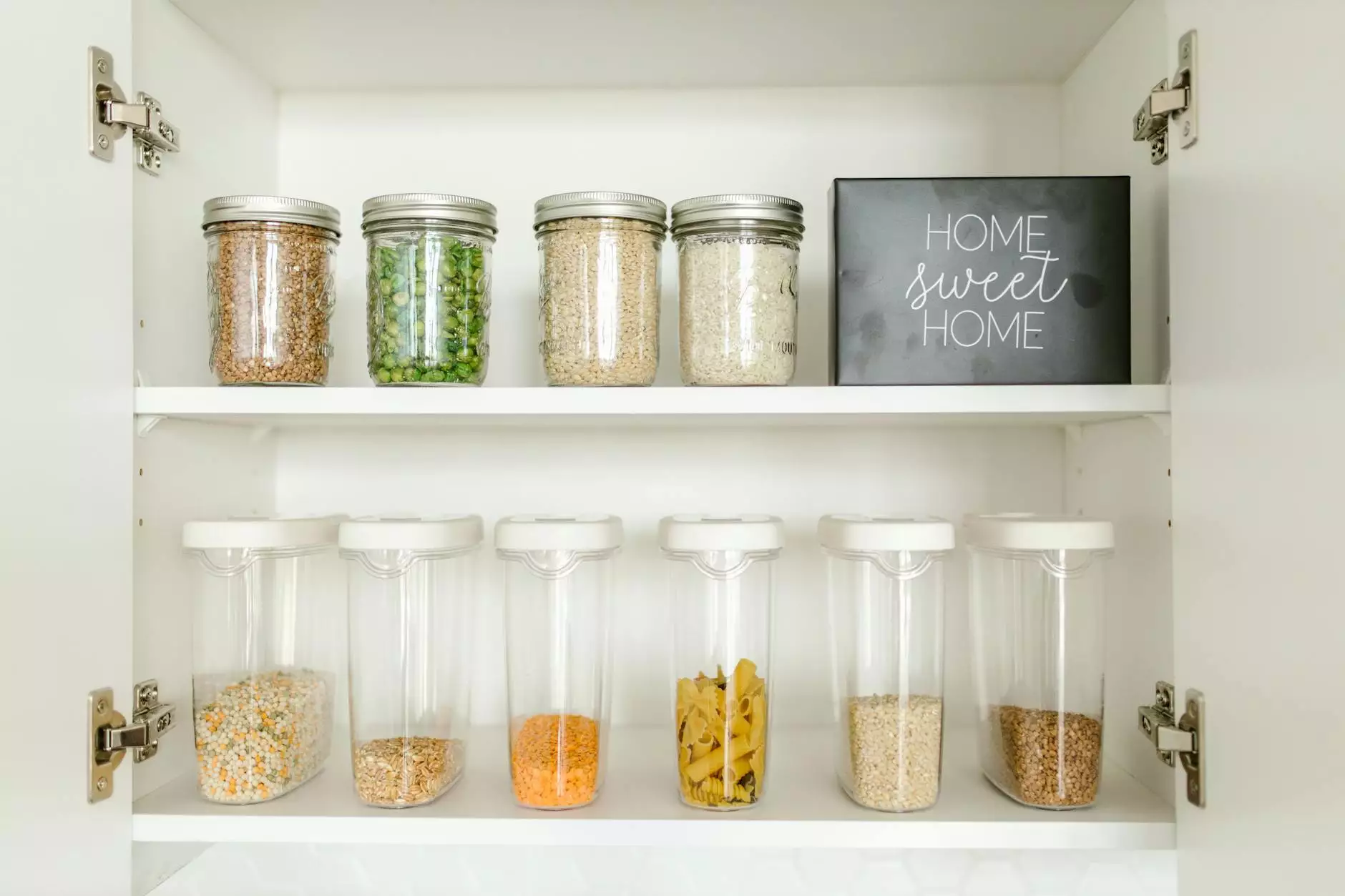
In the rapidly evolving field of artificial intelligence (AI), the importance of data labelling tools cannot be overstated. These tools serve as the backbone of machine learning algorithms by providing the essential infrastructure for data annotation. In this comprehensive article, we delve deep into the world of data labelling, exploring its significance, the various methodologies involved, and how businesses can leverage these tools effectively for superior outcomes.
What is Data Labelling?
Data labelling, in essence, is the process of identifying and tagging data with relevant information. This marked data is then used to train machine learning models to recognize patterns, make predictions, and automate tasks. Without accurate and efficient labelling, AI systems cannot function optimally, leading to degraded performance and inaccurate outcomes.
The Importance of Data Labelling Tools
The reliance on data labelling tools stems from several key factors:
- Accuracy: Precision is paramount in AI. Data labelling tools ensure that information is tagged correctly, which significantly enhances model performance.
- Scalability: As datasets grow, manually labelling can become unfeasible. Automated tools enable businesses to scale their data annotation efforts effortlessly.
- Efficiency: Utilizing advanced data labelling tools can reduce the time required for data preparation, accelerating project timelines and go-to-market strategies.
- Consistency: Consistent labelling across datasets is crucial for training robust AI models. Tools help maintain uniformity in data annotation, minimizing human error.
Types of Data Labelling Tools
With the increase in demand for data labelling, a variety of tools have emerged, each serving distinct purposes. Let’s examine the most popular types:
1. Image Data Labelling Tools
These tools specialize in annotating images, a requirement in fields such as computer vision. They allow users to label objects, identify boundaries, and count instances within images.
2. Text Data Labelling Tools
Essential for natural language processing (NLP), these tools help in tagging parts of speech, sentiment analysis, and topic categorization in textual data.
3. Audio Data Labelling Tools
Used in speech recognition, these tools annotate audio files, providing transcriptions, identifying speakers, and categorizing sounds.
4. Video Data Labelling Tools
For applications in surveillance, autonomous vehicles, and more, these tools allow for frame-by-frame annotation, making it easier to analyze moving images.
Key Features of a High-Quality Data Labelling Tool
Selecting the right data labelling tool can substantially impact the outcome of AI projects. Here are some crucial features to look for:
- User-friendly interface: An intuitive design enhances usability and minimizes the learning curve for new users.
- Collaboration tools: Features that allow teams to collaborate seamlessly can greatly improve workflow and efficiency.
- Integration capabilities: The ability to integrate with existing systems and workflows is vital for smooth operations.
- Automation options: Look for tools that offer automated labelling features to expedite the annotation process.
- Quality control mechanisms: Robust quality assurance processes ensure high levels of accuracy and reliability in the labelled data.
How KeyLabs.ai Sets the Standard in Data Labelling
KeyLabs.ai stands at the forefront of the data labelling tool market, offering a sophisticated platform that meets the diverse needs of businesses and developers alike. Here’s how:
Advanced Technology
By leveraging cutting-edge technologies such as machine learning algorithms and artificial intelligence, KeyLabs.ai enhances the labelling process, allowing for superior speed and accuracy. The tool continuously learns from user inputs, improving its performance over time.
Customization and Flexibility
Recognizing that every business has unique requirements, KeyLabs.ai provides customization options that enable organizations to tailor the labelling process to fit their specific use cases. This flexibility ensures that companies can adapt the platform as their needs evolve.
Comprehensive Support
KeyLabs.ai values customer satisfaction. Their dedicated support team is available to assist users with any questions or challenges they encounter, ensuring a smooth experience with the platform.
Benefits of Using a Professional Data Annotation Platform
Investing in a high-end data annotation platform like KeyLabs.ai brings numerous benefits to businesses:
- Improved Predictive Accuracy: High-quality labelled data leads to better-trained models which enhance the predictive accuracy of AI applications.
- Reduction in Time-to-Market: Efficient labelling practices allow businesses to bring products and services to market faster than competitors.
- Cost-Effectiveness: Outsourced or poorly executed labelling can be costly. Using a professional platform reduces errors and saves money in the long run.
- Enhanced Collaboration: Tools that facilitate teamwork lead to more effective project management and higher-quality outputs.
The Future of Data Labelling in Business
As AI technology advances, the demand for data labelling will only increase. Business leaders must prioritize investing in robust data labelling tools to keep pace with the competition. The landscape of data annotation is evolving, with trends indicating a greater reliance on automation and AI-driven solutions.
KeyLabs.ai is paving the way, adopting emerging technologies to refine and enhance the labelling process continually. As new innovations emerge, businesses that harness the power of advanced data annotation tools will not only stay relevant but will also excel in the marketplace.
Conclusion
In conclusion, the significance of data labelling tools in driving AI innovation cannot be ignored. By leveraging platforms like KeyLabs.ai, businesses can ensure they produce high-quality, accurately labelled datasets which are crucial for developing effective AI models. As the industry continues to grow, investing in the right data labelling strategy will become a defining factor for success.
Understanding the nuances of data labelling and evolving with the best practices and tools available is not just an option; it is a necessity for companies aiming to dominate their respective sectors in the age of AI.